Big data is often characterized by three (sometimes four or even five) V’s (e.g. Wedel and Kannan, 2016, Marr, 2016): Volume, Velocity, and Variety. More data was created in the past two years than in the entire previous history of mankind. At the same time, data is coming in at a much higher speed, often close to real-time. Furthermore, data nowadays is much more diverse, including not only numeric data but also text, images or video data to mention a few. The first two V’s are important from a storage and computational point of view, whereas the last V is important from an analytics point of view.
On the other hand, several people have argued that big data is just a hype that will go away. When we analyze the popularity of the search term “big data” on Google, we find that the usage of “big data” as search term has grown explosively since 2008, but has stabilized since about 2015 (figure 1). Marr (2016) states that the hype around “big data” and the name may disappear, but the phenomenon will stay and only gather momentum. He predicts that data will simply become the “new normal” in a few years’ time when all organizations use data to improve what they do and how they do it. We could not agree more with him.
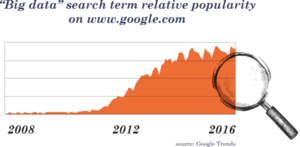
But, understanding and acting on an increasing volume and variety of data is not obvious. As Dan Ariely of Duke University once put it, “big data is like teenage sex: they all talk about it, they all want to do it, but no one really knows how to do it”. Wedel and Kanan (2016) put it more formally and argue that companies have invested too much in capturing and storing data and too little in the analytics part of it. While big data is on the top of many companies’ agenda’s, few of them are getting value out of it today. Therefore, in this special issue we did not only want to highlight “big data”, but also the “analytics” part of it, as state-of-the-art analytics is necessary to get results from big data.
We believe that a very basic lesson from “old-fashioned” marketing analytics also applies to the “new world” of big data, but is too often ignored: begin with an end in mind (Andreasen, 1985). If we do not know what decision we are trying to make, big data is not going to solve the problem: we are searching for the needle in a haystack without a needle. As Wedel and Kannan (2016, p115) note, the primary pre-condition for successful implementation of big data analytics is a culture of evidence-based decision making in an organization. Companies that are successful with big data analytics often have a C-level executive who oversees a data analytics center of excellence within the company. In such companies there prevails a culture of evidence-based decision making: instead of asking “what do we think?”, managers in such companies ask “what do we know?” or “what do the data say?” before making an important business decision. The big data analytics movement will also affect us at HEC Paris. We want to highlight three aspects, which are further discussed in this HEC Knowledge special issue.
First, it will affect the education and training of our students. In this special issue, the article by Daniel Brown discusses several initiatives at HEC Paris regarding the education of our students. Particularly, it highlights a new joint master with Polytechnique on big data integrating topics in statistics/econometrics, computer science, and substantive business areas such as marketing or finance. This new initiative will further extent the course offerings in business analytics that HEC Paris already has.
Second, it will increase the breath of our research. In this special issue, we highlight four recent studies to make that point. The first two studies by Peter Ebbes and Valeria Stourm discuss new developments in the context of marketing analytics. Their studies show how combining a variety of data sources benefits customer relationship management. The next two studies by Mitali Banerjee and Gilles Stoltz’ develop new machine learning algorithms to analyze pictures and to generate forecasts. These two studies show how such algorithms can be used to judge creativity and to aggregate forecasts to help businesses make better decisions. Lastly, David Restrepo Amariles and Xitong Li discuss several issues regarding big data legislation and policies. David shows that big data practices and algorithms are not always compliant with the rule of law, whereas Xitong argues that certain big data requirements in firm financial reporting can make financial reports actually more complex and harder to read.
Third, it will bring along opportunities for collaboration on problems of big data analytics between companies and researchers at HEC Paris. For empirical researchers at HEC Paris it is of increasing importance to be exposed to current business problems and data. At the same time, companies benefit from a close collaboration with researchers by getting access to state-of-the-art solutions and learning about the latest business analytic approaches in a field that is moving very rapidly. In fact, in several of our own past research studies, we have already successfully collaborated with companies on substantive data problems. Our collaborations have let to actionable insights for the company AND academic publications for the researcher(s), a clear WIN-WIN.
With this Knowledge@HEC special issue we highlight some of the exciting initiatives and research studies that are going on at HEC Paris. We hope that it inspires companies and alumni in the HEC Paris network to reach out to any of us with new data opportunities or big data analytics challenges, to further help to expand HEC Paris as a research institution!